AI amplifies human biases, and only human problem-solving can help to change this
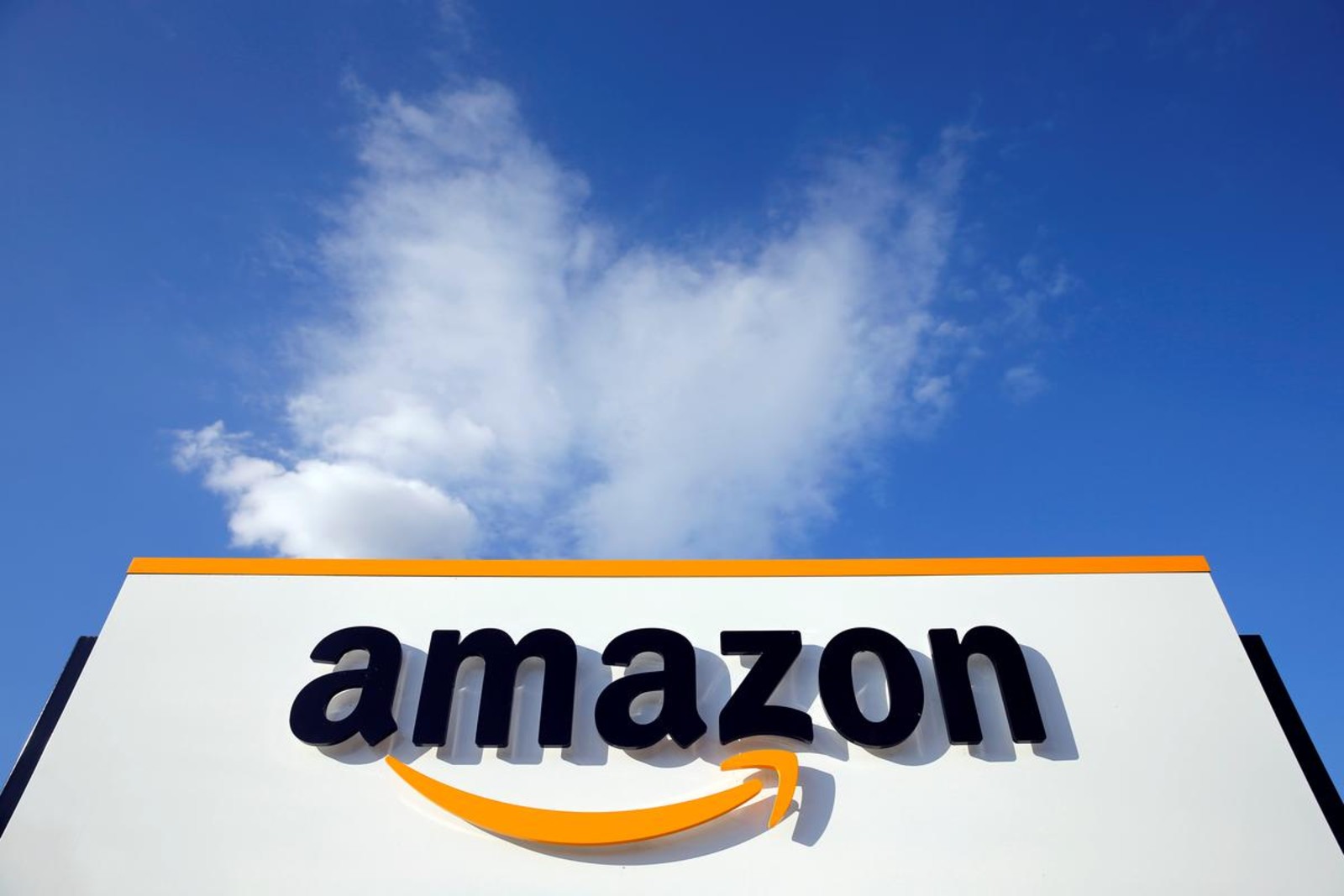
AI amplifies human biases, and only human problem-solving can help to change this. Algorithms have a powerful ability to mine data to find correlations at scale, but without the careful application of human insights as part of a continued process of learning, they will continue to reflect and amplify structural biases, theoretical neuroscientist Vivienne Ming writes in the Financial Times. Ming cites Amazon’s attempts to design an algorithm to promote fairer hiring as an example of this. The model was scrapped in 2015 because it was found to have embedded gender bias into its rating of candidates for technical positions, having been trained to observe and promote candidates based on patterns in resumes submitted to the company over a 10 year period — most of which came from men. Even when the company aimed to “de-bias” its data, the AI still discovered subtle patterns that distinguished male candidates from female, and overwhelmingly dismissed women applicants.
The problem is not biased data, but believing that an abundance of data can remove implicit biases. In fact, it is only by learning how to ask the right questions and applying human problem-solving skills (like the ability to observe and learn from failed solutions) to thorny issues like gender bias in hiring, that real progress can be made. A major trend in machine learning is training AI systems to focus on causal inference (asking “why”), and reinforcement-learning algorithms are an important move in that direction. But for now, Ming argues, the most effective way of using algorithms as helpful tools is by recognizing their limitations and focusing on training human problem solvers to ask the right questions in the first place.